Model may predict gMG disease symptoms’ response to treatment
Machine learning model shows whether symptoms will ease in 6 months
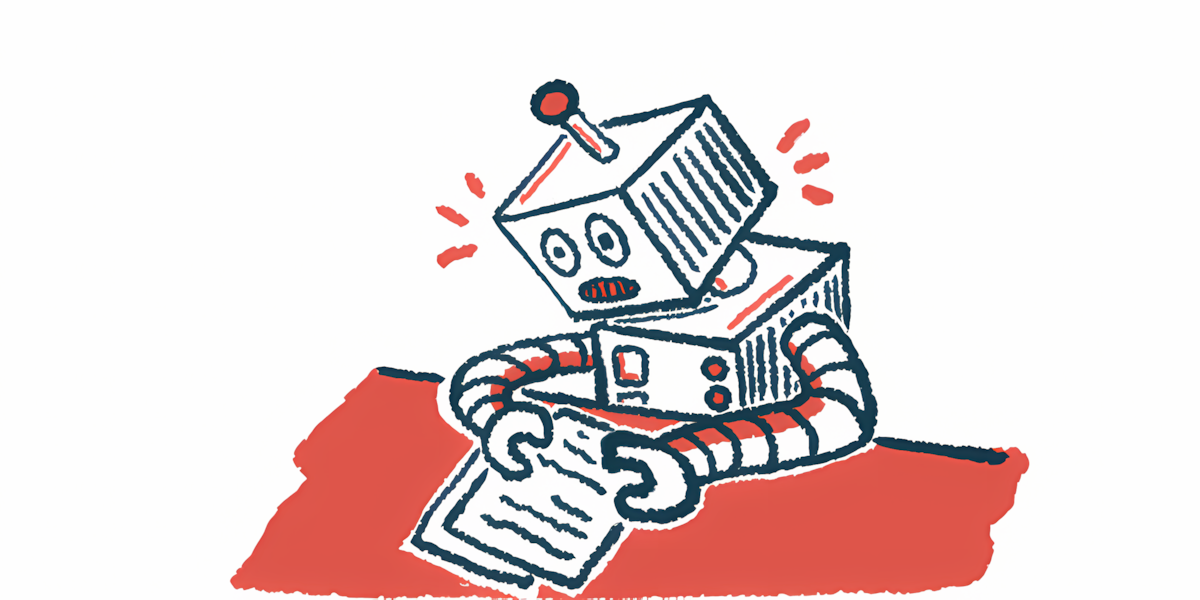
A machine learning model taking into account markers of inflammation and clinical data may accurately predict whether people with generalized myasthenia gravis (gMG) are likely to see their disease symptoms ease after six months of standard treatment.
Researchers described the new tool in the study, “Interpretable machine learning models for predicting short-term prognosis in AChR-Ab+ generalized myasthenia gravis using clinical features and systemic inflammation index,” published in Frontiers in Neurology.
MG is an autoimmune disease in which self-reactive antibodies disrupt communication between nerve and muscle cells, ultimately leading to disease symptoms of muscle weakness and fatigue. In the generalized form, muscles throughout the body are affected. A variety of treatments for MG are available, but they don’t work equally well in everyone with the disease, and it is difficult to predict which MG patients are most likely to benefit from standard therapy.
A team of scientists in China explored the use of machine learning to predict the short-term prognosis of people with gMG.
“Early prediction of treatment response facilitates tailored treatment strategies, potentially offering more intensive or alternative therapies to high-risk patients,” the researchers wrote. “Such strategies are likely to increase therapeutic success, slow disease progression, reduce hospital stays, and enhance patient quality of life.”
Data from 202 patients with common antibody
Machine learning is a branch of artificial intelligence in which a battery of data is fed into a computer, alongside a set of mathematical rules that the computer uses to identify patterns in the data. The computer can then apply these patterns to make sense of new data.
The researchers used data from 202 people with gMG who were positive for antibodies targeting the acetylcholine receptor (AChR), the most common type of MG-causing antibody.
All patients were treated with pyridostigmine, a standard MG treatment sold under the brand name Mestinon. Some were also given the corticosteroid prednisone and/or the immunosuppressant tacrolimus.
To evaluate outcomes, researchers divided patients into two groups: a good outcome group consisting of patients who saw their disease symptoms ease after six months of treatment, and the poor outcome group, those in whom symptoms remained unchanged or worsened. Just under three-quarters of the patients saw their symptoms ease.
The researchers used data from about two-thirds of the patients to train the machine learning program. The remaining data was then used to test the program.
To test the model’s accuracy, the researchers calculated the area under the receiver operating characteristic curve, or AUC. This is a mathematical test of how accurately the model can distinguish between two groups, in this case, patients who did or did not benefit from treatment. AUC values range from 0.5 to 1, with higher values reflecting better accuracy.
The best performing machine learning model in this study yielded an AUC of 0.944. This model took into account clinical data, such as disease duration and standardized measures of MG severity, as well as data on several inflammation markers.
“This research signifies a substantial advancement in using [machine learning] to integrate clinical indicators with the systemic inflammation index for accurate short-term prognosis assessments,” the scientists wrote, adding that the study “highlights the capability of [machine learning] models that integrate clinical indicators and the systemic inflammation index to predict the short-term prognosis of [AChR-positive] gMG patients.”
The investigators noted that further verification and validation will be needed to confirm the utility of this new machine learning tool.