Recording Eye Movements May Help Diagnose MG Earlier, Study Suggests
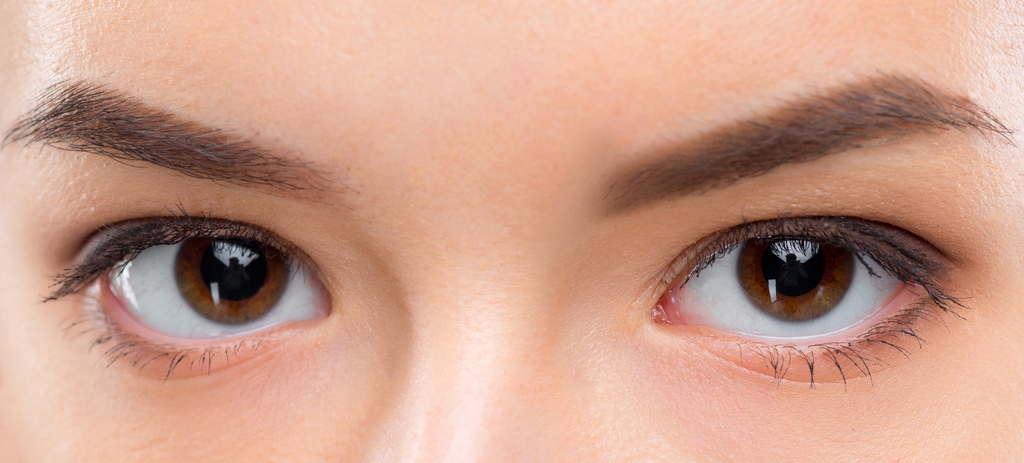
Assessing altered eye movements with a technique called electrooculography (EOG) may become a non-invasive method for early diagnosis of myasthenia gravis (MG), according to new research.
The study, “Analysis of Electrooculography Signals for the Detection of Myasthenia Gravis,” was published in the journal Clinical Neurophysiology.
The most used electrophysiological (electrical activity) methods — single-fiber electromyography and repetitive nerve stimulation — to diagnose MG comes with limitations, such as causing discomfort for patients and requiring special training for diagnosticians.
Though not currently used in clinical practice, EOG is an alternative that can be used to measure changes in eye movements, which are early symptoms in a significant proportion of MG patients that progress to the generalized form of the disease.
Based on a previous study, a team from the University of Toronto and Ryerson University sought to assess in the new study whether analysis of EOG signals around the eyes could help screen for MG. The data were collected from eight patients (age was not published in the study) with mild to moderate MG and 31 unaffected controls during the wake stage in two sleep tests — multiple sleep latency test and overnight polysomnography.
To gauge eye movements, the scientists placed one electrode 1 cm below the left outer canthus (the corner of the eye) and another electrode placed 1 cm above the right outer canthus. To increase efficiency, they analyzed only the initial 10 minutes of the seven hours of data obtained during the sleep tests.
The researchers used two signal processing techniques — time and wavelet (small waveforms) — and developed an algorithm to detect eye movement. They focused on slow eye movements since MG induces muscle fatigue.
The results of the time domain approach revealed 82.1%–83.3% accuracy, 62.5%–68.8% sensitivity, and 87.1% specificity, when considering each sleep study separately or combining their data.
In addition, using the wavelet domain approach led to an 82.1%–87.2% accuracy, 87.5% sensitivity, and 80.6%–87.1% specificity.
“Our results demonstrate that an EOG-based signal analysis is a potentially viable non-invasive alternative for MG screening,” the scientists wrote, adding that “regardless of the sleep test used, up to 10 minutes could be sufficient to achieve acceptable diagnostic accuracy and specificity.”
“The proposed approach could lead to early detection of MG and thereby improve clinical outcomes in this population,” they added.